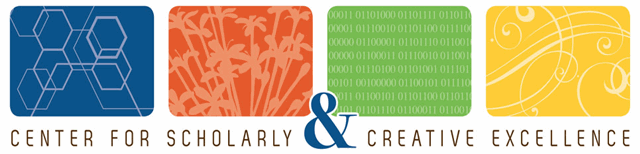
Faculty Scholarly Dissemination Grants
The Use of Stochastic Resonance to Improve Detectability in CT Images
Department
School of Engineering
College
Padnos College of Engineering and Computing
Date Range
2014-2015
Disciplines
Engineering
Abstract
General education is more than a list of required courses a student must take to complete their degree. For most universities, general education is the groundwork for the student's university experience. These courses span multiple disciplines and allow students to experience a wide range of topics on their path to graduation. Programming classes, e.g., Introduction to Programming, have not typically been an option as part of a general education course sequence at most universities. This study found that, only half of universities offer any kind of programming course in the General Education (GenEd) Program. The data also show that only two-thirds of institutions offer a computing class of any kind as a general education option. Institutions with ABET accredited Information Systems (IS) programs are significantly lower in both of these categories. This paper demonstrates the reasoning and process for including a programming class as an option in a GenEd Program, thereby showing how a programming class can be used to satisfy the requirements of a general education course. This results in two significant advantages to the computing department and university since the departments expand their reach to many more students, with a potential of increasing the number of majors or minors within the department; and non-computing majors have the opportunity to take courses that have not traditionally been offered in the GenEd Program. The latter results in students receiving a more comprehensive education and exposure to skills in high demand. Introduction: In non-linear threshold based systems characterized by one or two stable states, the transition from one state to another is caused by a signal exceeding a specified threshold. Some input signals of very low amplitude may not cross this threshold and therefore will not be detected. The addition of a low-amplitude, sub-threshold stochastic signal can allow the original signal to exceed threshold and thus be detected [1]. This process of adding low amplitude white noise to reveal underlying patterns in a signal is called stochastic resonance and has been applied to enhancing images from low-field MRI scanners [2]. We apply static noise models of SR to CT images and hypothesize that using SR techniques will lead to improved diagnosability from these images. Materials and Methods: All software was written in MATLAB. Simulations were performed on test images that consists of oval shapes with small variations in amplitude (A) that are indistinguishable when observed on a standard laptop screen (B). First, a 12-bit test image mimicking the standard CT image bit resolution was created. This image was converted to an 8-bit image for viewing on a standard laptop computer screen. So any differences in pixel intensity in the 12-bit image were now under the quantization threshold in the 8-bit images thus preventing the oval shapes from being discernible. Next, an image, the same size as the test image, of normally distributed, zero-mean, white Gaussian noise was generated as follows: A compound image consisting of the spatially added test and noise images was computed and evaluated to see if the sub-threshold elements were discernible. Noise variance values between 2x10-6 and 3x10-5 were iteratively tested [3] in their ability to produce an SR effect and improve the visualization of sub-threshold intensities. Results: Figure C shows the result of iteratively adding white noise to the test image in increasing values of variance. Note that the oval shapes are now more distinguishable but the background noise has stayed relatively constant. This would not have been the case if a simple amplification technique had been used. A B C Conclusions: Preliminary results suggest that SR may be a valuable tool for improving the readability of CT images. The next steps include investigating the effects of dynamic windowing for producing an SR effect, and implementing this technique on clinical CT images so radiologists can evaluate its efficacy for improving CT image diagnosabilty. References: [1]. B. Andò and S. Graziani, Stochastic Resonance: Theory and Applications. Springer, 2000, p. 220. [2]. V. P. S. Rallabandi and P. K. Roy, Magnetic resonance image enhancement using stochastic resonance in Fourier domain., Magn. Reson. Imaging, vol. 28, no. 9, pp. 1361 73, Nov. 2010. [3]. J. Yeh, W. Hsu, Y.-C. Chang, M.-T. Lo, and Y.-H. Lin, Application of stochastic resonance for imaging enhancement of computed tomography in hepatocellular carcinoma, in 2011 IEEE International Conference on Bioinformatics and Biomedicine Workshops (BIBMW), 2011, pp. 9459 47.
Conference Name
Annual Meeting of the Biomedical Engineering Society
Conference Location
San Antonio, Texas
ScholarWorks Citation
Rhodes, Samhita; Zweifel, Nadina; Strahle, Roland; Scheidegger, Stephan; Fuchslin, Rudolf; and Rhodes, Samhita, "The Use of Stochastic Resonance to Improve Detectability in CT Images" (2015). Faculty Scholarly Dissemination Grants. 468.
https://scholarworks.gvsu.edu/fsdg/468